2021. 3. 13. 18:43ㆍ카테고리 없음
용어
asymmetric distortions: 비대칭 외곡
distort the sensor streams asymmetrically : 센서 데이터를 비대칭적으로 비틀다, 외곡하다
intertwine : 뒤얽히다 , 엮이다
인트로
좋은 날씨에 biased 되어 학습되었다.
[Existing object detection methods, including efficient Single Shot detectors (SSD) [41], are trained on auto motive datasets that are biased towards good weather con ditions. While these methods work well in good condi tions [19, 59], they fail in rare weather events (top). Lidar only detectors, such as the same SSD model trained on pro jected lidar depth, might be distorted due to severe backscat ter in fog or snow (center). ]
[While these existing methods, and the autonomous system that performs decision making on their outputs, perform well under normal imaging conditions, they fail in adverse weather and imaging conditions. This is because
existing training datasets are biased towards clear weather conditions, and detector architectures are designed to rely only on the redundant information in the undistorted sensory streams. ]
fusion 이유 / contribution
제안된 방법(하단)은 이러한 드문 시나리오의 훈련 데이터를 보지 않고 멀티모달 데이터에서 보이지 않는(잠재적으로 비대칭) 왜곡을 처리하는 방법을 학습한다.
[The proposed method (bottom) learns to tackle unseen (potentially asymmetric) distortions in multimodal data without seeing training data of these rare scenarios]
Specifically, we make the following contributions:
• We introduce a multimodal adverse weather dataset
covering camera, lidar, radar, gated NIR, and FIR sensor data. The dataset contains rare scenarios, such as
heavy fog, heavy snow, and severe rain, during more
than 10,000 km of driving in northern Europe.
• We propose a deep multimodal fusion network which
departs from proposal-level fusion, and instead adaptively fuses driven by measurement entropy.
• We assess the model on the proposed dataset, validating that it generalizes to unseen asymmetric distortions. The approach outperforms state-of-the-art fusion methods more than 8% AP in hard scenarios independent of weather, including light fog, dense fog,
snow, and clear conditions, and it runs in real-time.
이 연구에서는 큰 주석된(annotated) 학습 데이터 없이 특이 상황에 대해 발견한다.
In this work, we propose a multimodal fusion method for
object detection in adverse weather, including fog, snow,
and harsh rain, without having large annotated training
datasets available for these scenarios.
연구 내용에 대한 정리 (in intro)
we propose an adaptive single-shot deep fusion architecture which exchanges features in intertwined feature extractor blocks. This deep early fusion is steered by measured entropy. The proposed adaptive fusion allows us to learn models that generalize across scenarios. To validate our approach, we address the bias in existing datasets by introducing a novel multimodal dataset acquired on three months of acquisition in northern Europe
안개 상황에 대한 인트로
thick fog is observable only during 0.01 % of typical driving in North America, and even in foggy regions, dense fog with visibility below 50 m occurs only up to 15 times a year [62].
안개는 자주 발생안한다는 reference
안개는 자주 발생안하고 엄청 rare 하다
[On the roles of circulation and aerosols in the decline of mist anddense fog in Europe over the last 30 years]
많은 데이터 중에 정말 적다
adverse weather-distorted data are underrepresented in general Moreover, existing methods are limited to image data but not to multisensor data, e.g. including lidar point-cloud data.
[66] H. Xu, Y. Gao, F. Yu, and T. Darrell. End-to-end learning of
driving models from large-scale video datasets. In Proceedings of the IEEE Conference on Computer Vision and Pattern
Recognition, pages 2174–2182, 2017. 2, 3 ]
[59] P. Sun, H. Kretzschmar, X. Dotiwalla, A. Chouard, V. Patnaik, P. Tsui, J. Guo, Y. Zhou, Y. Chai, B. Caine, V. Vasudevan, W. Han, J. Ngiam, H. Zhao, A. Timofeev, S. Ettinger,
M. Krivokon, A. Gao, A. Joshi, Y. Zhang, J. Shlens, Z. Chen,
and D. Anguelov. Scalability in perception for autonomous
driving: Waymo open dataset, 2019. 1, 2, 3]
존재하는 fusion 방법 또한 TD 의 bias 로 인하여 센서의 비틀어짐에 대해서 고민하지 않는다.
Existing fusion methods have been proposed mostly for
lidar-camera setups [65, 11, 43, 36, 12], as a result of the
limited sensor inputs in existing training datasets [66, 19,
59]. These methods do not only struggle with sensor distortions in adverse weather due to the bias of the training data.
카메라 라이다는 특정상황에서 성능이 안좋다(backscatter , low-light scene)
In rain and snow, small particles affect the color image and lidar depth estimates equally through backscatter.
Adversely, in foggy or snowy conditions, state-of-the-art
pulsed lidar systems are restricted to less than 20 m range
due to backscatter,
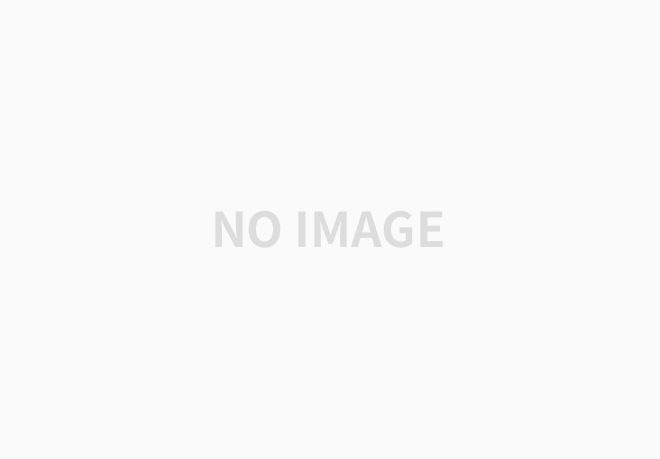
진행된 연구들
1. 안개등 데이터를 제거 후 인지 프로세스 진행
A large body of
work explores methods for the removal of sensor distortions before processing.
2. 전통적인 intensity 이미지 데이터에서 fog , haze 를 제거하는것은 광범위하게 진행되었다.
Especially fog and haze removal
from conventional intensity image data have been explored
extensively [68, 71, 34, 54, 37, 7, 38, 47].
3. 안개 결과는 contrast 와 color 데이터에서 거리 종속적 손실이 있다.
Fog results in a distance-dependent loss in contrast and color.
4. 안개 제거 방법은 display 용도로만 제안된다. / 또한 다운스트림 sematic 작업의 성능을 향상시키기 위한 사전 처리로 제안되었다 [52].
Fog removal methods have not only been suggested for display application.
it has also been proposed as preprocessing to improve the performance of downstream semantic
tasks